By Atharva Chilwarwar
In recent times, the Indian banking sector has embarked on an unwavering journey of incorporating AI-enabled technologies into their operations, ushering in a new era of innovation and efficiency. According to the PwC Fin-Tech Trends Report of 2017, the investment in AI and its allied technologies in the sector is projected to surpass a staggering $5.5 billion, rising significantly from $4.1 billion in 2015. LLMs are no longer just a concept or an experiment. They’re increasingly playing a critical role in our digital landscape. To delve deeper into this paradigm shift, it becomes imperative to comprehend the essence of Large Language Models (LLMs) and their strategic implementation in the Banking, Financial Services, and Insurance (BFSI) sector.
The world of language technology is buzzing with excitement, and for good reason. The natural language processing (NLP) market is on a rocket-like trajectory, projected to skyrocket from $11 billion in 2020 to an astonishing $35 billion by 2026. But it’s not just the market figures that are ballooning; the actual language models themselves are growing too, both in size and smarts.
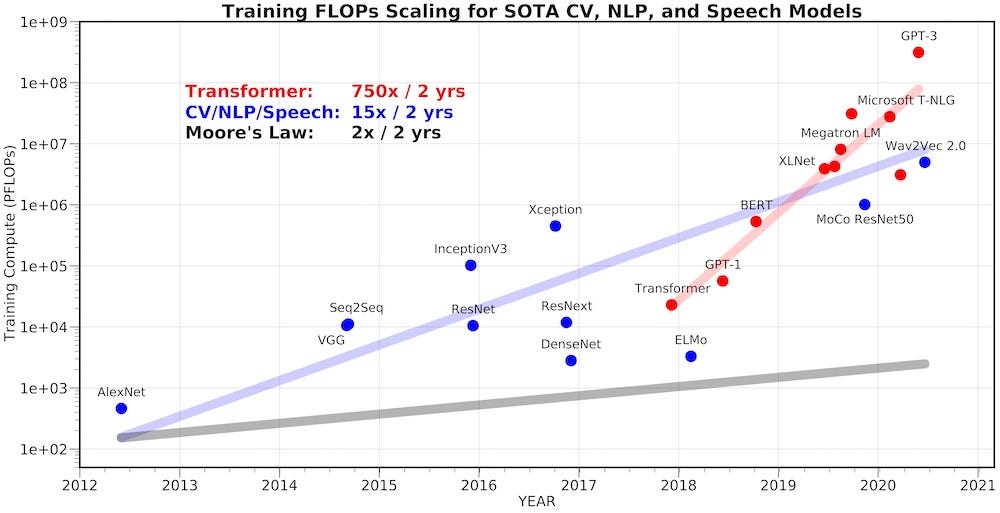
Understanding Large Language Models (LLMs)
Large Language Models, or LLMs, represent a class of advanced artificial intelligence systems designed to interpret, generate, and interact with human-like text. These systems are founded on deep learning techniques and are meticulously trained on massive datasets comprising billions of words from diverse sources, such as websites, books, and articles. This extensive training equips LLMs with an unparalleled grasp of language intricacies, grammar rules, contextual nuances, and even certain facets of general knowledge.
To gain a profound understanding of LLMs, several key concepts deserve recognition:
1. Word Embedding: The process of translating words into a numerical format interpretable by AI models, facilitating seamless linguistic comprehension.
2. Transformer Mechanism: This innovation empowers models to concurrently weigh and consider all aspects of input data, eliminating the need for sequential processing. It addresses a common challenge in natural language processing—managing long-range dependencies in text.
3. Attention Mechanism: These sophisticated components help the AI model prioritize certain elements within the input text over others when generating an output.
4. Fine Tuning: After initial training on vast datasets, models can undergo further refinement or ‘fine-tuning’ using more specific datasets, optimizing their performance for particular tasks.
5. Bias Mitigation: LLMs, being products of their training data, can inadvertently inherit biases present in that data. Ethical development of LLMs requires a rigorous approach to recognize and mitigate such biases.
Prominent LLMs, exemplified by OpenAI’s GPT-3, employ neural networks called transformers, which empower them to adeptly tackle intricate language-related tasks.
Role of LLMs in the BFSI Sector
In the context of India, a burgeoning Fin-Tech industry is poised to grow at an impressive Compound Annual Growth Rate (CAGR) of 22% in the next five years, with a projected business volume of $73 billion by 2020. The accessibility of the internet to over half a billion individuals in the country accentuates the potential of the Fin-Tech sector. Notably, financial services are reaching more than 300 million consumers, granting them access to credit, a segment often overlooked by conventional players due to limited credit histories.
Utilization of LLMs in BFSI
LLMs tailored for the banking domain possess multifaceted capabilities that align seamlessly with the industry’s demands, thanks to their ability to comprehend and generate language in a remarkably human-like manner. Given the vast and diverse financial data landscape, LLMs are uniquely poised to deliver substantial value to businesses within the sector. The integration of LLMs, including GPT-4, BERT, RoBERTa, and specialized models like BloombergGPT, has shown promise in transforming various aspects of the fintech landscape.
Key Applications of LLMs in Banking
1. Fraud Detection and Prevention: LLMs equipped with AI prowess prove invaluable in real-time analysis of extensive financial data, enhancing the detection of fraudulent activities. This added layer of security bolsters customer confidence and safeguards the reputation of banks.
2. Risk Assessment and Management: LLMs excel in informed decision-making by analyzing diverse data sources, thereby mitigating potential risks.
3. Personalized Customer Service: AI-driven chatbots and virtual assistants, empowered by LLMs, usher in a new era of highly tailored customer experiences.
4. News Analysis and Sentiment Detection: LLMs adeptly analyze news headlines, earnings reports, social media content, and other sources, facilitating trend identification and sentiment analysis.
5. Portfolio Management LLMs: Extend invaluable support to investment-focused banks by analyzing market trends and offering portfolio allocation recommendations, thereby optimizing investment strategies for customers.
Conclusion
In this inaugural segment of our blog series, we lay the foundation for an enlightening exploration. Brace yourself for an insightful journey, as in the subsequent instalment, we delve into the remarkable realm of AlgoFabric. Prepare to witness the convergence of cutting-edge technology and ingenuity, culminating in the creation of an exceptional Software as a Service (SaaS) platform.
The impending sequel promises an in-depth revelation of how AlgoAnalytics has ingeniously harnessed the power of sentiment analysis through the ingenious employment of Latent Lexical Semantic Mapping (LLMS). Anticipate a comprehensive dissection of the mechanisms underpinning this groundbreaking technology, as we unveil the intricate web of algorithms that drive its core functionalities.
Stay tuned for Part II
Our upcoming instalment will unravel the threads connecting the dots between AlgoAnalytics ingenious conception, the mechanics of LLMs, and the holistic tapestry of components that constitute this state-of-the-art SaaS platform. Join us as we navigate the labyrinthine corridors of sentiment analysis, shedding light on each facet with clarity and precision. Your understanding of this revolutionary technology is about to transcend boundaries, so stay tuned for a transformative revelation that promises to redefine your perspective on analytics and its real-world applications.