By Atharva Chilwarwar
The rapid advancements in Large Language Models (LLMs) have ushered in a new era of recommendation systems, introducing us to the world of Recommendation via LLM, or Recommended Large Language Models for short. In this blog, we embark on a comprehensive exploration of the crucial issue of fairness within the context of Recommended Large Language Models. Although LLMs have proven their mettle in various tasks, it is imperative to recognize the potential presence of social biases and assess the fairness of recommendations generated by Recommended Large Language Models. In this in-depth analysis, we will delve into the nuances of this emerging recommendation paradigm, highlighting its unique challenges and proposing solutions.
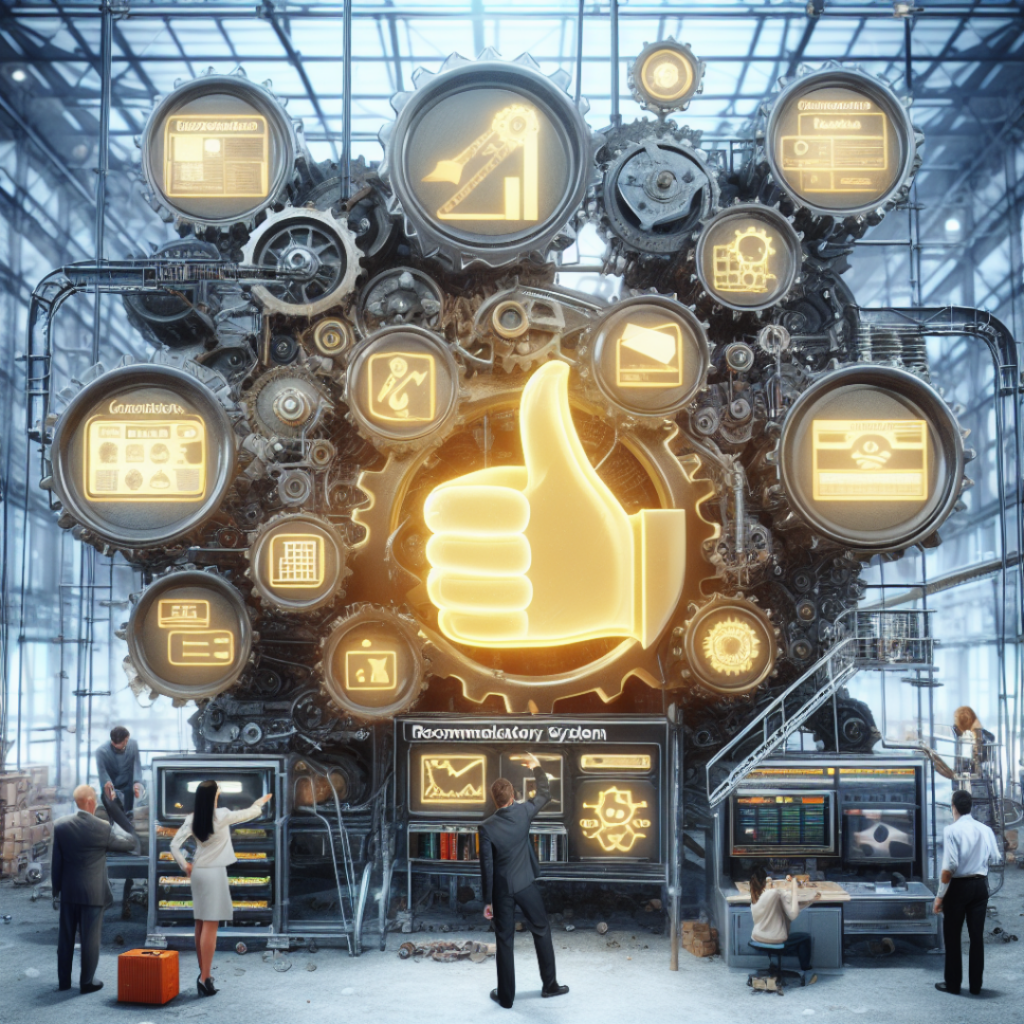
Section 1: The Emergence of Recommended Large Language Models
The world of recommendations is evolving at a breathtaking pace, largely thanks to the capabilities of LLMs. These models are like digital wizards, capable of understanding and generating human-like text. They’ve not only revolutionized how we interact with information but have also given birth to a novel recommendation paradigm: Recommended Large Language Models.
Understanding Recommended Large Language Models
Recommended Large Language Models represent a paradigm shift in how recommendations are made. In contrast to traditional systems, which provide suggestions based on past user behaviour, Recommended Large Language Models engage in a dynamic conversation with the user. Users can ask questions or make requests, and Recommended Large Language Models respond by generating recommendations based on these inputs. It’s like having a conversation with an intelligent assistant that tailors its responses to your needs.
For instance, imagine asking Recommended Large Language Models for song recommendations. You could simply say, “Give me 20 song titles, please,” and Recommended Large Language Models would craft a list of 20 song titles for your enjoyment. The possibilities are endless, and the potential for personalized recommendations is exciting. However, this flexibility also brings about new challenges, particularly in the realm of fairness.
Section 2: The Fairness Conundrum
One of the key concerns with Recommended Large Language Models is the potential for unfair recommendations. How can a model that learns from vast and diverse data sources, including text from the internet, ensure that its recommendations are free from biases and prejudices?
The Unseen Biases
LLMs learn from the text they are exposed to, and the vast amount of data on the internet contains a multitude of perspectives, including some that are unfair, biased, or even harmful. As a result, LLMs can inadvertently perpetuate these biases when generating recommendations. This becomes a problem because the recommendations produced by Recommended Large Language Models have the potential to influence decisions and actions, impacting individuals and communities.
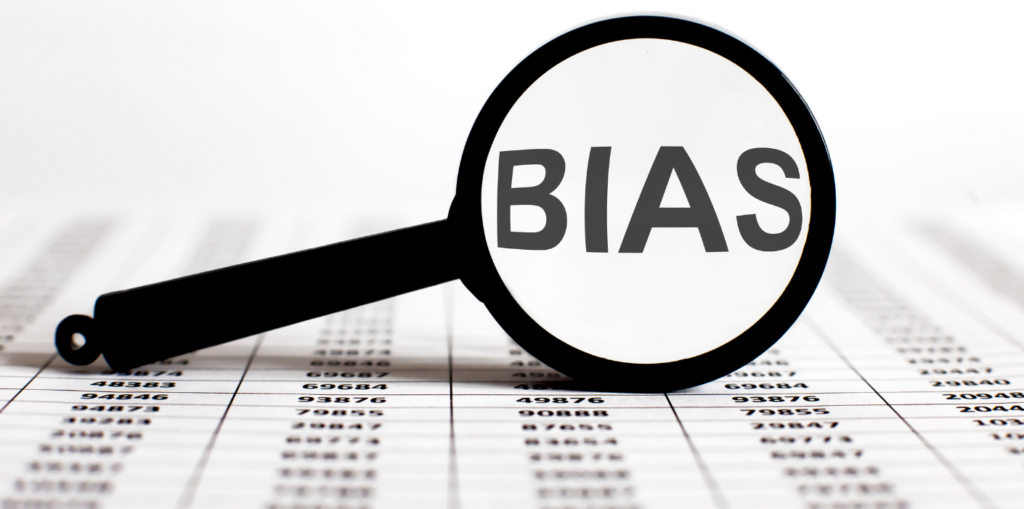
Fairness Matters
Fairness is a paramount concern, not only for Recommended Large Language Models but for recommendation systems in general. Ensuring fairness is essential because these systems wield immense influence in various aspects of our lives, from product recommendations to content discovery and more. There is a rich history of research on fairness in traditional recommendation systems, but the unique characteristics of Recommended Large Language Models call for a fresh perspective.
Section 3: Exploring Fairness in Recommended Large Language Models
In this section, we dive into the heart of the matter – fairness in Recommended Large Language Models. We’ll discuss how personal attributes, privacy concerns, and the challenges of measuring fairness pose unique problems for this emerging recommendation paradigm.
User Attributes and Fairness
In the Recommended Large Language Models landscape, some users may opt not to disclose certain personal attributes, like their race or skin colour, due to privacy concerns. While this privacy protection is important, it can introduce a fairness challenge. Why? LLMs, based on their training data, may have inherent preferences or biases, even if you don’t explicitly provide that information.
When users withhold these attributes, the recommendations they receive can become skewed, unintentionally favouring or disadvantage in particular user groups. This can lead to unfairness, particularly for groups that are already vulnerable or underrepresented. Addressing this issue is critical for ensuring that Recommended Large Language Models benefits all users equally.
Challenges in Measuring Fairness
Measuring fairness in Recommended Large Language Models presents a unique set of challenges. Traditional fairness measurement methods often require access to model prediction scores, which can be challenging to obtain in the context of Recommended Large Language Models. Additionally, these methods typically operate on a fixed set of candidates based on specific datasets, which is not ideal for the dynamic and adaptable nature of Recommended Large Language Models.
Section 4: Addressing Fairness in Recommended Large Language Models
The research community has recognized the importance of fairness and is actively working on solutions to mitigate bias and promote fair recommendations. Here, we discuss some of these promising developments.
New Benchmarks for Fairness
To better evaluate the fairness and harmfulness of LLMs, the research community has introduced innovative benchmarks. Examples include CrowS-Pairs, a dataset with sentence pairs that highlight stereotyping, and RealToxicityPrompts and RedTeamingData, which contain prompts that could lead to harmful or toxic responses. HELM offers a holistic evaluation of large language models, considering both bias and fairness.
Section 5: A Gap in Research
Despite the considerable progress in the field of natural language processing (NLP), there remains a noticeable gap in our understanding of the fairness of Recommended Large Language Models. The extensive research on fairness in LLMs has not been fully extended to this emerging recommendation paradigm. This is the impetus for our work.
A Call to Explore Fairness in Recommended Large Language Models
Our research aims to bridge this gap by initiating an exploration of fairness in Recommended Large Language Models. We intend to shed light on the unique fairness challenges posed by this innovative recommendation paradigm, providing a foundation for further investigation and progress in this critical area.
Conclusion
In this blog, we’ve journeyed through the intricate landscape of Recommended Large Language Models and fairness. We’ve seen how the capabilities of LLMs have opened up new frontiers in recommendations, but these advancements come with their own set of challenges. Ensuring fairness in Recommended Large Language Models is crucial, especially when it has the potential to impact individuals and communities.
As we navigate this uncharted territory, it’s clear that addressing fairness in Recommended Large Language Models requires innovative solutions. The research community is already making significant strides in this direction, with promising approaches to reduce bias and benchmarks that help us evaluate fairness more effectively. Despite its challenges, it’s imperative to explore fairness within this emerging paradigm to ensure that Recommended Large Language Models can benefit all users without perpetuating biases or causing harm.
References
https://arxiv.org/abs/2305.07609
https://arxiv.org/abs/2305.12090